In 2020-2021, two Fellows were selected: Prajna Soni and Sara Alanis. The fellowship is extremely successful, illustrated by the fact that the first CITIES fellows got accepted to the University of California Berkeley and MIT to pursue their studies.
Enhancing Urban Seawall Sustainability Using Ecological Engineering
Fellow: Sara Alanis
Supervisor: Kemal Celik and John Burt
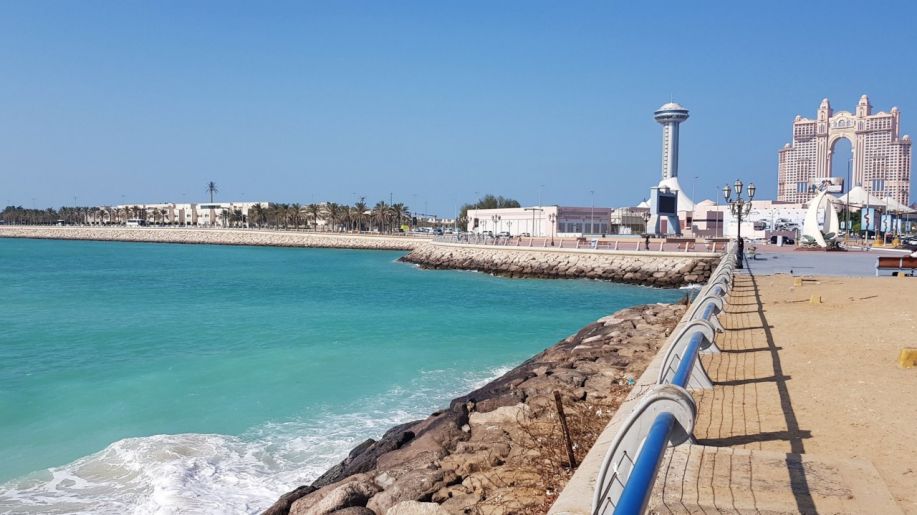
The aim of this multidisciplinary study is to develop approaches to optimize the material and texture of concrete seawall bricks that are being increasingly used to protect cities against heavy waves, sea storms, and rising sea levels in order to enhance the diversity and abundance of intertidal organisms. Natural shorelines are increasingly replaced with the artificial infrastructure needed to support growing economies and populations. This coastal urban development brings additional pressures on the marine fauna inhabiting the coastal zone. In this study, the changes in the intertidal inhabitant’s coverage and composition over time are tracked to understand which treatment (material and surface texture) best enhances marine biodiversity. This might potentially help to develop more sustainable urban shorelines that mitigate the ecological effects of coastal urban infrastructure and strengthen local biodiversity.
Do Recommender Systems Learn Our Preferences or Guide Them?
Fellow: Prajna Soni
Supervisor: Saif Eddin Jabari
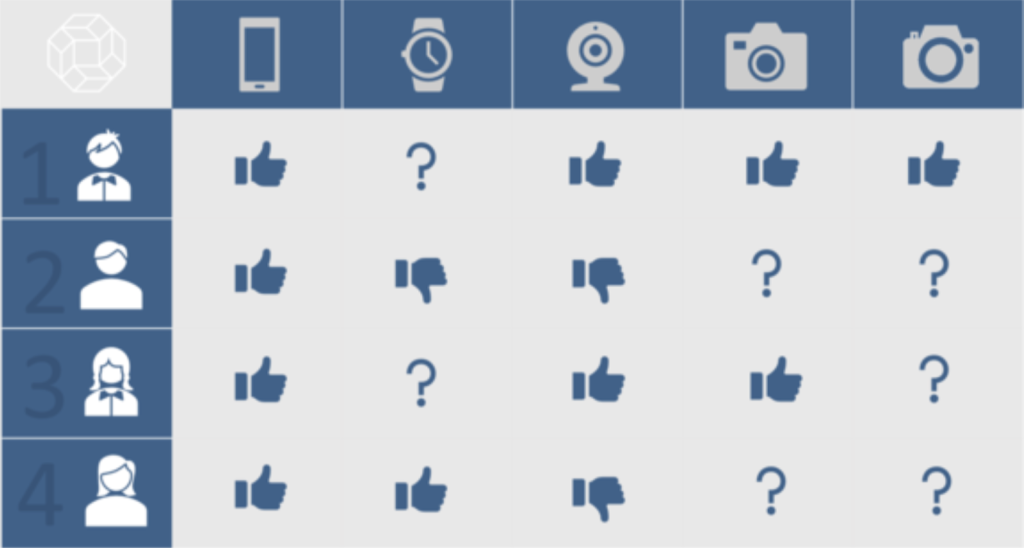
Recommender systems are algorithms that govern everything we see online, from search results on search engines to online shopping to video streaming. To produce recommendations, they rely on our input (e.g., search/shopping history and ratings), but they also use information from other users to create our recommendations. This allows the biases of other users to influence what we see. This project investigates the evolution of user classes as a result of interaction with a recommender system. We utilize both real data made public by a popular streaming service and synthetic data. We investigate the impact that biased users have on the recommendations made to “common” users. Ultimately, this research asks whether biased users can systematically influence what we see and to what extent.